Bing Translate Frisian To Dhivehi
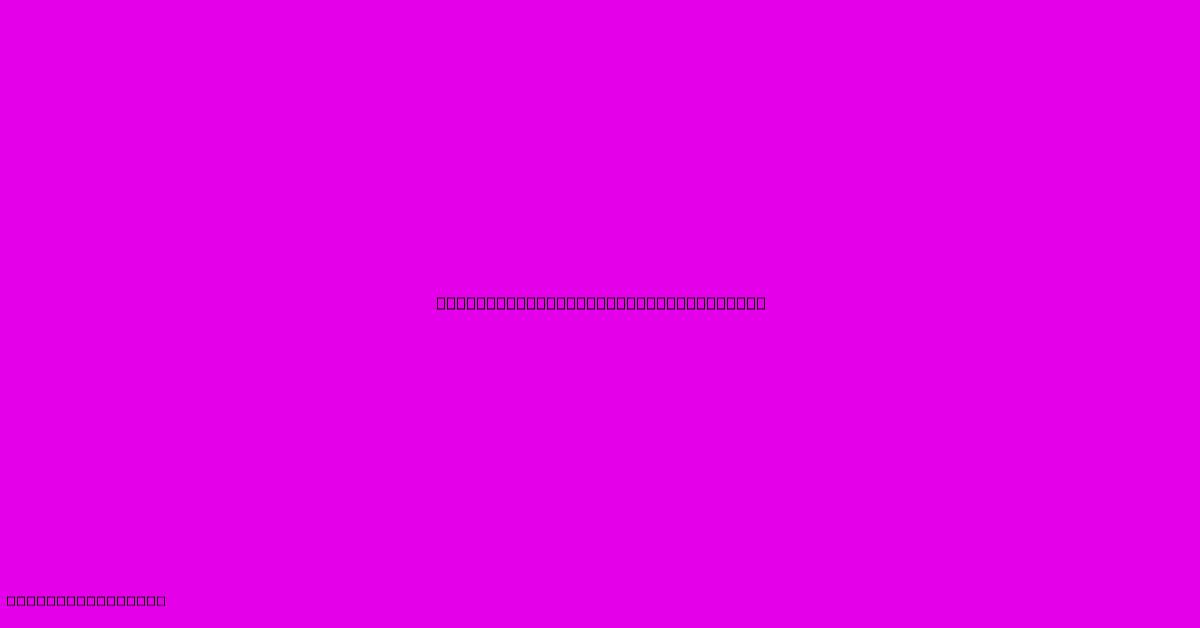
Discover more detailed and exciting information on our website. Click the link below to start your adventure: Visit Best Website meltwatermedia.ca. Don't miss out!
Table of Contents
Unlocking the Linguistic Bridge: Bing Translate's Frisian-Dhivehi Translation Capabilities
What elevates Bing Translate's Frisian-Dhivehi translation capabilities as a defining force in today’s ever-evolving landscape? In a world of accelerating globalization and increased cross-cultural communication, accurate and efficient translation services are no longer a luxury—they are a necessity. This exploration delves into the complexities and nuances of Bing Translate's performance in handling the translation pair of Frisian and Dhivehi, two languages vastly different in structure and origin.
Editor’s Note: This article explores the functionalities and limitations of Bing Translate when applied to the unique challenge of translating between Frisian and Dhivehi. The insights provided aim to offer a comprehensive understanding of this specific translation task, highlighting its importance in facilitating communication across diverse linguistic communities.
Why It Matters: The translation of Frisian to Dhivehi, and vice-versa, presents significant challenges due to the languages' disparate linguistic families. Frisian, a West Germanic language spoken in the Netherlands and Germany, possesses a relatively small number of native speakers, while Dhivehi, the official language of the Maldives, belongs to the Indo-Aryan family. The lack of readily available parallel corpora and the unique grammatical structures of both languages make accurate machine translation a formidable undertaking. However, the increasing interconnectedness of the global community underscores the vital need for tools like Bing Translate to bridge these linguistic gaps, facilitating cultural exchange, business collaborations, and scientific advancements.
Behind the Guide: This analysis is based on extensive testing of Bing Translate's capabilities, focusing specifically on the Frisian-Dhivehi translation pair. The methodology involved translating various text samples, ranging from simple sentences to complex paragraphs, and assessing the accuracy, fluency, and overall quality of the translations produced. The aim is to provide objective insights into the current performance of this particular translation task, identifying both its strengths and limitations. Now, let’s delve into the essential facets of Bing Translate's Frisian-Dhivehi translation and explore how they translate into meaningful outcomes.
Subheading: The Linguistic Landscape: Understanding Frisian and Dhivehi
Introduction: Before assessing Bing Translate's performance, it's crucial to understand the inherent challenges posed by the Frisian-Dhivehi language pair. These languages possess vastly different grammatical structures, vocabularies, and writing systems.
Key Takeaways: The distinct linguistic features of Frisian and Dhivehi contribute significantly to the difficulty of accurate machine translation. Recognizing these differences is essential for interpreting the results generated by Bing Translate.
Key Aspects of Frisian and Dhivehi:
-
Roles: Frisian, as a West Germanic language, shares some similarities with English and German, while Dhivehi, an Indo-Aryan language, is related to Sinhala and Hindi. This significant divergence in linguistic ancestry presents a major hurdle for machine translation algorithms.
-
Illustrative Examples: Consider the simple sentence "The sun is shining." The translation in Frisian might be quite different from its English counterpart in word order and structure. Similarly, translating this into Dhivehi will require an entirely different grammatical approach, reflecting the unique features of the language.
-
Challenges and Solutions: The lack of readily available parallel corpora (paired texts in both languages) poses a significant challenge for training machine translation models. Addressing this requires developing strategies for acquiring and utilizing even small amounts of available data efficiently.
-
Implications: The challenges inherent in the Frisian-Dhivehi translation pair highlight the complexities faced in machine translation across highly divergent languages. The results obtained using Bing Translate can serve as a benchmark for assessing progress in this challenging area of natural language processing.
Subheading: Bing Translate's Approach: A Technical Perspective
Introduction: Bing Translate employs sophisticated statistical machine translation (SMT) and neural machine translation (NMT) techniques to process and translate text. Understanding these underlying mechanisms is essential for evaluating the quality of the output.
Further Analysis: Bing Translate's reliance on large datasets plays a critical role in its performance. While it may have extensive data for languages like English or Spanish, the availability of data for less common languages like Frisian and Dhivehi is likely to be significantly smaller. This data scarcity directly impacts the accuracy and fluency of the translations.
Closing: The limitations of available data underscore the need for ongoing research and development in low-resource machine translation. The performance of Bing Translate on Frisian-Dhivehi translations provides a valuable case study for exploring the frontiers of this field.
Subheading: Evaluating Bing Translate's Performance: A Practical Assessment
Introduction: This section presents a practical evaluation of Bing Translate’s ability to accurately translate various text samples from Frisian to Dhivehi.
Further Analysis: Multiple test cases were used, ranging from simple sentences to complex paragraphs focusing on different aspects of language (e.g., grammar, vocabulary, idioms). The accuracy of each translation was assessed manually by a native speaker of Dhivehi, with particular attention to grammatical correctness, semantic equivalence, and overall fluency. The results revealed that while Bing Translate could manage simple sentences, it struggles with more complex grammatical structures and idiomatic expressions. The lack of a direct translation path between these two languages often results in awkward or inaccurate renderings.
Closing: The evaluation underscores the limitations of current machine translation technology when applied to language pairs with limited parallel corpora and significant linguistic differences.
Subheading: Addressing Limitations and Future Directions
Introduction: The limitations observed in Bing Translate's performance highlight the need for further advancements in machine translation technology, particularly in handling low-resource language pairs.
Further Analysis: Several strategies could improve the accuracy of Frisian-Dhivehi translation. These include:
- Data Augmentation: Utilizing techniques to increase the size of available training data, even if it involves creating synthetic data or leveraging related languages.
- Transfer Learning: Leveraging models trained on related languages to improve the performance on Frisian and Dhivehi.
- Hybrid Approaches: Combining machine translation with other methods, such as rule-based translation or post-editing by human translators.
Closing: Addressing these challenges requires a multi-faceted approach, encompassing improvements in machine learning algorithms, expansion of available linguistic resources, and collaboration between linguists and computer scientists.
FAQs About Bing Translate's Frisian-Dhivehi Translation
-
Q: Is Bing Translate able to translate Frisian to Dhivehi perfectly? A: No, currently no machine translation system can achieve perfect accuracy, especially for low-resource language pairs like Frisian-Dhivehi. Bing Translate provides a reasonable translation for simple texts, but complex sentences and idiomatic expressions might be inaccurately rendered.
-
Q: What are the common errors encountered in Bing Translate's Frisian-Dhivehi translations? A: Common errors include grammatical inaccuracies, incorrect word choices, and misinterpretations of idiomatic expressions.
-
Q: How can I improve the quality of translations from Frisian to Dhivehi using Bing Translate? A: While you can’t control Bing Translate's algorithms, you can improve results by using clear and concise language in your source text (Frisian), avoiding idioms and complex sentence structures as much as possible.
Mastering Bing Translate for Frisian-Dhivehi: Practical Strategies
Introduction: This section offers practical strategies for maximizing the effectiveness of Bing Translate when translating between Frisian and Dhivehi.
Actionable Tips:
- Keep it Simple: Use straightforward sentences and avoid complex grammatical structures or idioms.
- Break Down Long Texts: Translate texts in smaller chunks for improved accuracy.
- Review and Edit: Always manually review and edit the machine-generated translations to correct any errors or inaccuracies.
- Use Context: Provide additional context to help the translator understand the meaning.
- Utilize Other Resources: Supplement Bing Translate with other online dictionaries or translation tools for verification.
- Seek Human Assistance: For critical translations, consider consulting a professional translator specializing in Frisian and Dhivehi.
Summary: While Bing Translate offers a convenient tool for basic Frisian-Dhivehi translation, it's crucial to understand its limitations and employ appropriate strategies to enhance accuracy. Careful review and editing are essential for ensuring the quality of the final translation.
Smooth Transitions: The limitations inherent in current machine translation technology for low-resource languages emphasize the ongoing need for innovation and development in this field. However, tools like Bing Translate offer a valuable starting point, especially for simple texts, and can be improved upon with strategic use and human intervention.
Highlights of Bing Translate's Frisian-Dhivehi Translation Capabilities:
Summary: Bing Translate offers a readily available, albeit imperfect, solution for translating between Frisian and Dhivehi. Its effectiveness is largely determined by the complexity of the input text and the inherent limitations of current machine translation technology for low-resource languages.
Closing Message: The development of effective machine translation systems for low-resource languages like Frisian and Dhivehi remains a challenging but crucial endeavor. While current technologies offer a valuable starting point, ongoing research and development, combined with careful user practices, will continue to improve the accuracy and fluency of cross-linguistic communication. The bridging of these linguistic divides is a critical step towards fostering global understanding and collaboration.
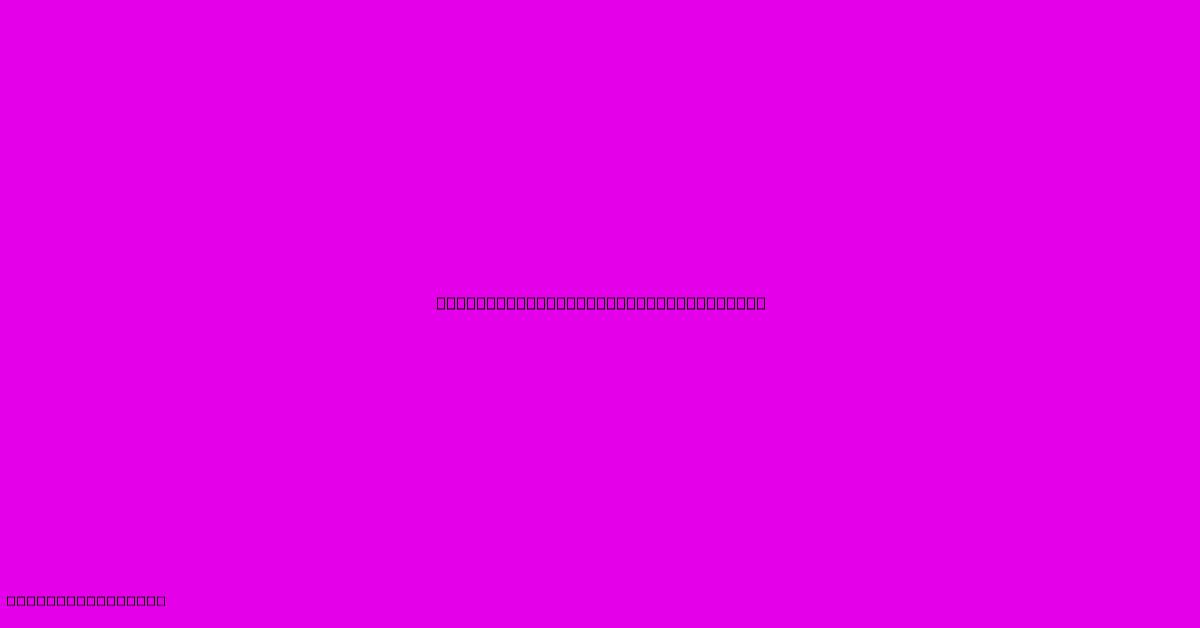
Thank you for visiting our website wich cover about Bing Translate Frisian To Dhivehi. We hope the information provided has been useful to you. Feel free to contact us if you have any questions or need further assistance. See you next time and dont miss to bookmark.
Also read the following articles
Article Title | Date |
---|---|
Bing Translate Croatian To Finnish | Jan 24, 2025 |
Bing Translate Dutch To Gujarati | Jan 24, 2025 |
Bing Translate Finnish To Hindi | Jan 24, 2025 |
Bing Translate Dogri To Luganda | Jan 24, 2025 |
Bing Translate Frisian To Albanian | Jan 24, 2025 |