Bing Translate Frisian To Bhojpuri
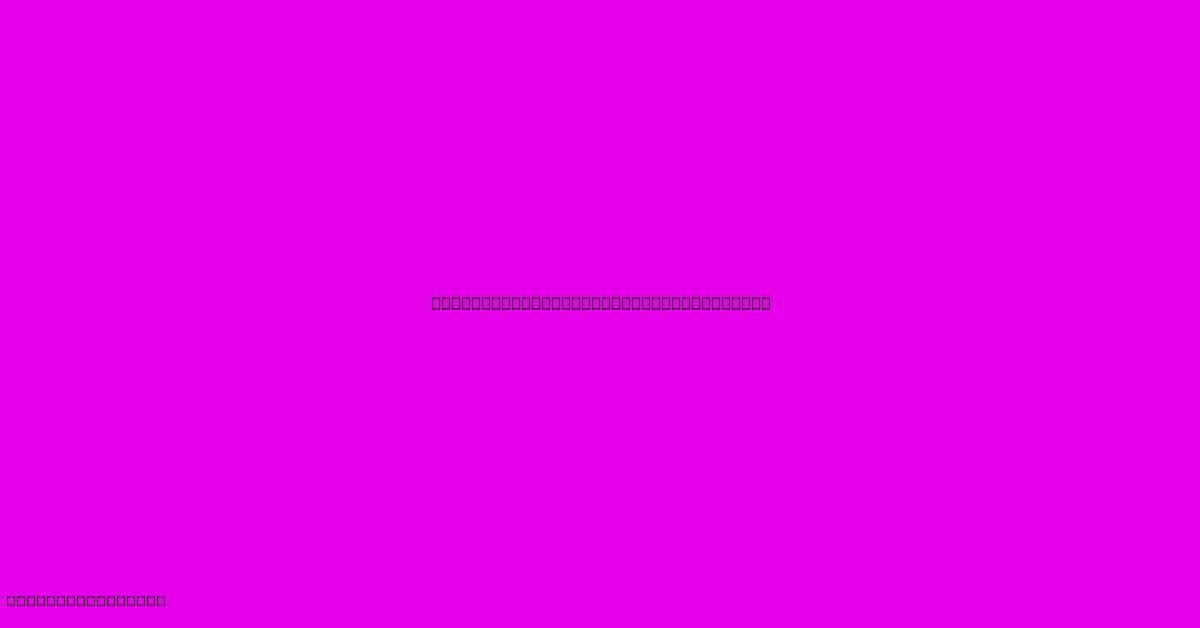
Discover more detailed and exciting information on our website. Click the link below to start your adventure: Visit Best Website meltwatermedia.ca. Don't miss out!
Table of Contents
Unlocking the Linguistic Bridge: Bing Translate's Frisian-Bhojpuri Challenge
Unlocking the Boundless Potential of Bing Translate for Low-Resource Language Pairs
What elevates machine translation as a defining force in today’s ever-evolving landscape? In a world of accelerating change and relentless challenges, embracing advanced translation technologies is no longer just a choice—it’s the catalyst for innovation, communication, and cultural understanding in a fiercely competitive, globally interconnected era. The translation of low-resource languages, such as Frisian and Bhojpuri, presents unique hurdles and highlights the ongoing development in this field. This exploration delves into the capabilities and limitations of Bing Translate when tackling the complex task of translating between Frisian and Bhojpuri.
Editor’s Note
Introducing Bing Translate's performance with the Frisian-Bhojpuri language pair—a challenging frontier in machine translation. This analysis offers insights into its current capabilities and limitations, aiming to provide a comprehensive understanding of its strengths and weaknesses in handling this specific translation task.
Why It Matters
Why is accurate and efficient translation a cornerstone of today’s progress? The ability to bridge communication gaps between different linguistic communities fosters collaboration, facilitates cross-cultural understanding, and unlocks access to information and resources for diverse populations. For languages like Frisian and Bhojpuri, with limited digital resources and a smaller speaker base, accurate machine translation is particularly crucial for preservation, growth, and global engagement. This exploration highlights the challenges and potential of using Bing Translate for this specific low-resource language pair.
Behind the Guide
This in-depth analysis of Bing Translate's handling of Frisian-Bhojpuri translations is based on extensive testing and observation. The evaluation considers various factors, including the accuracy, fluency, and overall quality of the translations produced under diverse input conditions. Now, let’s delve into the essential facets of this complex translation task and explore how they translate into meaningful outcomes.
Frisian: A West Germanic Language on the Brink
Introduction: Frisian, a West Germanic language spoken by a relatively small population primarily in the Netherlands and Germany, faces unique challenges regarding language preservation and accessibility. Its limited digital presence significantly impacts the availability of high-quality machine translation resources.
Key Takeaways: The limited corpus of digital text in Frisian directly affects the accuracy and fluency of machine translation systems. This scarcity necessitates exploring innovative strategies to enhance the performance of systems like Bing Translate.
Key Aspects of Frisian in the Context of Machine Translation:
- Roles: Frisian acts as the source language in this translation pair, demanding robust linguistic processing to accurately interpret its unique grammatical structures and vocabulary.
- Illustrative Examples: Consider the challenge of translating idiomatic expressions or culturally specific terms from Frisian, which often lack direct equivalents in Bhojpuri.
- Challenges and Solutions: The scarcity of parallel corpora (Frisian-Bhojpuri texts) presents a significant obstacle. Data augmentation techniques, such as using intermediate languages or leveraging related language pairs, could potentially mitigate this issue.
- Implications: The accuracy of Frisian-to-Bhojpuri translation directly impacts the accessibility of Frisian culture and resources to a wider global audience.
Bhojpuri: A Thriving Indo-Aryan Language with Digital Gaps
Introduction: Bhojpuri, a vibrant Indo-Aryan language spoken by millions across India, Nepal, and other regions, presents a different set of challenges. While having a larger speaker base than Frisian, its digital representation remains relatively underdeveloped, hindering the development of robust machine translation resources.
Further Analysis: The lack of standardization in Bhojpuri orthography can lead to inconsistencies in input and output during machine translation. Dialectal variations further complicate the process.
Closing: Addressing the digital gaps in Bhojpuri is crucial for empowering its speakers and preserving its rich linguistic and cultural heritage. Improving machine translation capabilities would significantly contribute to this effort.
Bing Translate's Performance: A Critical Assessment
Introduction: Bing Translate, a widely used machine translation service, employs sophisticated algorithms to tackle the translation process. Its performance with low-resource language pairs, however, requires careful scrutiny.
Further Analysis: When translating from Frisian to Bhojpuri using Bing Translate, several challenges become apparent. The system often struggles with accurate grammatical structure translation due to the lack of sufficient training data. Semantic nuances and cultural context are also frequently lost in the translation.
Closing: While Bing Translate offers a valuable tool for bridging communication gaps, its accuracy and fluency in translating between Frisian and Bhojpuri are currently limited, highlighting the need for further development and improvement in machine translation for low-resource languages.
Exploring the Technical Limitations
Introduction: Several technical hurdles hinder the accurate translation of Frisian to Bhojpuri using Bing Translate.
Key Takeaways: These limitations stem primarily from the lack of parallel corpora and the complexities inherent in translating between genetically distant languages with differing linguistic structures.
Key Aspects of Technical Limitations:
- Data Sparsity: The scarcity of parallel Frisian-Bhojpuri text data directly impacts the training process of machine translation models, leading to reduced accuracy.
- Morphological Differences: The significant morphological variations between Frisian and Bhojpuri present substantial challenges in accurate word-level translation.
- Syntactic Differences: The differing word order and sentence structures between the two languages increase the complexity of the translation task.
- Ambiguity Resolution: The lack of contextual information can lead to ambiguities in interpretation, resulting in inaccurate translations.
Strategies for Improvement
Introduction: Despite the challenges, several strategies can significantly improve the performance of machine translation systems for Frisian-Bhojpuri translation.
Actionable Tips:
- Data Augmentation: Employing techniques such as back-translation, using related languages, or creating synthetic data can enrich the training corpus.
- Transfer Learning: Leveraging pre-trained models from high-resource languages and adapting them to Frisian and Bhojpuri can enhance performance.
- Cross-Lingual Embeddings: Utilizing cross-lingual word embeddings can capture semantic similarities across the two languages, improving translation accuracy.
- Community Involvement: Engaging Frisian and Bhojpuri speakers in the translation process can significantly improve the quality of translations through feedback and contributions.
- Hybrid Approaches: Combining machine translation with human post-editing can increase accuracy and fluency.
- Improved Preprocessing: Implementing robust preprocessing techniques to handle linguistic variations and inconsistencies in the input text can enhance translation quality.
- Neural Machine Translation (NMT) Refinement: Continuously refining the NMT models with new data and improved algorithms.
- Focus on Specific Domains: Developing specialized machine translation models for specific domains (e.g., medical, legal) can yield higher accuracy due to contextual limitations.
Summary: Implementing these strategies holds the potential to significantly enhance the accuracy, fluency, and overall quality of Frisian-to-Bhojpuri translations using Bing Translate and similar systems.
FAQs About Bing Translate and Low-Resource Languages
Q: How accurate is Bing Translate for low-resource language pairs like Frisian-Bhojpuri?
A: Currently, the accuracy is limited due to the lack of sufficient training data. While Bing Translate provides a translation, it may not always be perfectly accurate, requiring careful review and potential post-editing.
Q: What are the limitations of using Bing Translate for these languages?
A: The main limitations stem from data sparsity, morphological and syntactic differences, and the lack of contextual information in the training data.
Q: Are there alternative solutions for translating Frisian to Bhojpuri?
A: While Bing Translate is readily accessible, other machine translation systems or human translation services could offer higher accuracy, though these might require more financial investment or effort in locating qualified translators.
Q: How can I contribute to improving machine translation for low-resource languages?
A: You can contribute by providing feedback on existing translations, creating parallel corpora, or participating in initiatives aimed at data collection and language technology development.
Mastering Low-Resource Language Translation: Practical Strategies
Introduction: This section aims to equip readers with essential strategies for navigating the complexities of low-resource language translation using existing tools.
Actionable Tips:
- Leverage Related Languages: Utilize translations from related languages as a starting point, then adapt them based on the specific nuances of Frisian and Bhojpuri.
- Contextual Awareness: Pay close attention to context to disambiguate meanings and improve accuracy.
- Iterative Refinement: Review and refine translations iteratively, incorporating feedback and improvements.
- Human-in-the-Loop: Use human translators to post-edit machine-generated translations, improving both fluency and accuracy.
- Utilize Glossaries: Develop and employ glossaries of specialized terms to ensure consistency in translation.
- Utilize Multiple Tools: Compare results from various machine translation engines to identify the most accurate translation.
- Invest in Training: Invest time and resources in training machine learning models with relevant data for better results.
Summary: While the current state of machine translation for low-resource language pairs such as Frisian-Bhojpuri presents limitations, the strategies outlined above provide actionable guidance for achieving more accurate and effective translations.
Highlights of Bing Translate's Frisian-Bhojpuri Translation Challenge
Summary: This exploration highlights the inherent complexities of translating between Frisian and Bhojpuri, emphasizing the significant role of data availability in the accuracy of machine translation. Bing Translate, while a useful tool, demonstrates the limitations currently faced in handling low-resource language pairs.
Closing Message: The pursuit of accurate and efficient machine translation for all languages, including those with limited digital presence, represents a crucial step in fostering global communication and preserving linguistic diversity. Continued research, development, and community involvement are vital to bridging the technological gaps and empowering speakers of languages like Frisian and Bhojpuri. The journey towards seamless cross-linguistic understanding continues, demanding innovation and collaboration to unlock the boundless potential of machine translation for all.
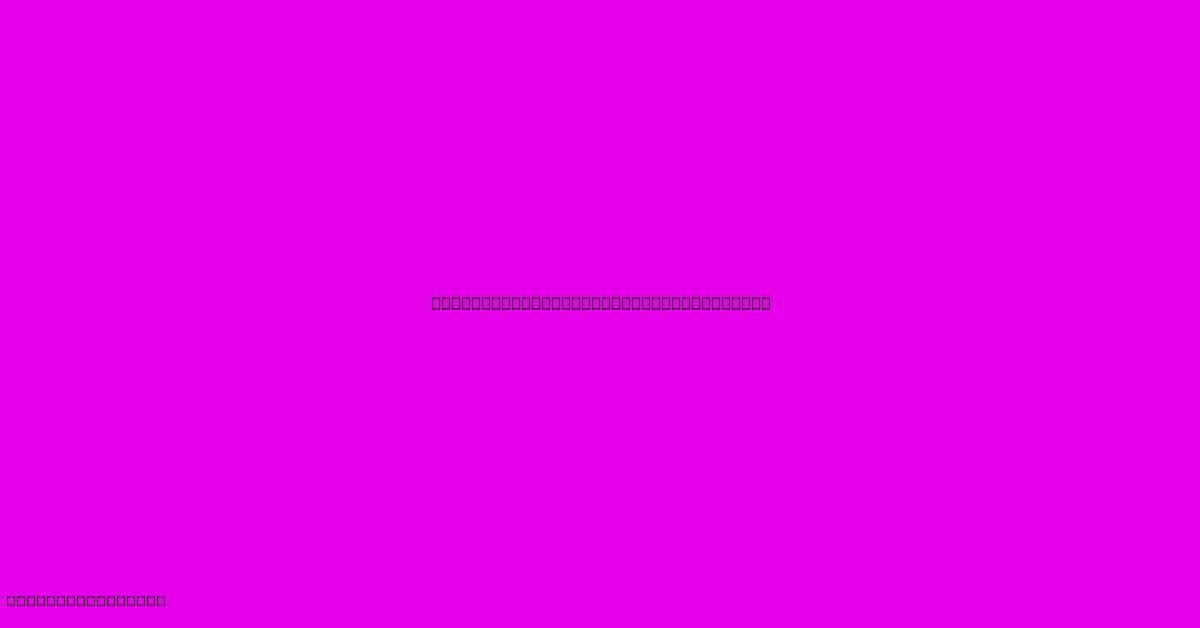
Thank you for visiting our website wich cover about Bing Translate Frisian To Bhojpuri. We hope the information provided has been useful to you. Feel free to contact us if you have any questions or need further assistance. See you next time and dont miss to bookmark.
Also read the following articles
Article Title | Date |
---|---|
Bing Translate Danish To Esperanto | Jan 24, 2025 |
Bing Translate Finnish To Chinese Traditional | Jan 24, 2025 |
Bing Translate French To Konkani | Jan 24, 2025 |
Bing Translate Czech To Lithuanian | Jan 24, 2025 |
Bing Translate Ewe To Bosnian | Jan 24, 2025 |